Data is the crude oil of the technology-driven world, as a result, data analytics and data science have become the new buzzwords. According to IT chronicles, around 2 Quintillion bytes of data are being generated daily across all industries. Traditional data processing systems can not manage a large amount of data. However, handling big data can be solved with data science and data analytics. These terms sound similar, and people think these terms are interchangeable, creating confusion.
This article will cater to the data science vs. data analytics discussion and bring out various factors that will make it easy to detect which field is more suitable for you.
What is Data Science?
Data science is a study that deals with big data to design and build various processes for data modeling, analysis, and prediction workflows. It uses machine learning algorithms to create, train, and build predictive models that help analyze data. A report in 2020 by MicroStrategy stated that about 94% of businesses agree that data is essential for business growth. However, 63% of companies cannot gather insights, which is how data science has found its place in many industry domains, such as marketing, sales, healthcare, finance, and more.
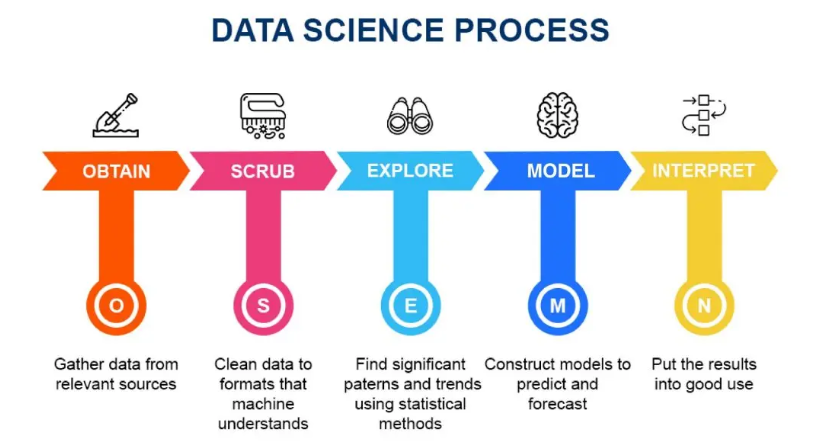
The data science lifecycle revolves around five stages — obtaining, scrubbing, exploring, modeling, and interpreting data. Obtaining data involves gathering raw unstructured data from different sources through various methods like data extraction and data entry. After collecting is the scrubbing or cleaning procedure, where the transformation of raw data into clean and structured information. Once the data is clean, data scientists process and explore it to determine its purpose. Data undergoes text mining, prediction, and qualitative analysis. The final step in the data science lifecycle is interpretation, which involves data reporting via BI (business intelligence) and data visualization.Â
The prerequisites for data science are machine learning and statistics. Machine learning is the spine of data science, as it is vital for quality predictions and computations. You can train and automate models to make more intelligent decisions to save time and effort through machine learning. To master machine learning, knowing statistics is essential and is an integral part of data science. With mathematical statistics, you can interpret quantitative data and better understand the correlations between different attributes of algorithms. To implement machine learning and statistics, a data scientist should know programming languages like Python or R and understand database management concepts like extracting or filtering data using basic SQL commands.
What is Data Analytics?
If data science is a house, then data analytics would be a room in that house. Data analytics is the process of exploring existing structured raw data with a specific goal in mind. It finds hidden patterns, new trends, and correlations to derive essential insights. Data analytics has many uses, such as decision-making, customer experience, operations, and marketing strategies.
The main steps in data analytics are understanding the problem, data cleaning, data enhancement, data exploration, and visualization of the result. Understanding the issue is the first and most crucial step as it defines the goals and areas where work is required. Once you identify the problem, data relevant to it needs to be collected and filtered. The disordered raw data needs cleaning and should be void of redundant, missing, and unwanted values. After gathering the processed data, exploration and analysis using business intelligence tools and data visualization take place to understand the data and figure areas to improve.
For data analytics, the prerequisites are Microsoft Excel, SQL, presentation skills, and machine learning. A basic understanding of Excel is fundamental as data analytics majorly involves many spreadsheets, but Excel is not efficient for more extensive datasets. SQL or Structured Query Language is essential for a data analyst as one can easily manage massive datasets. Knowledge of machine learning and software languages like R or Python is also beneficial when dealing with big data. After getting the desired results from analytics, demonstrating the idea in a simple but engaging way is crucial. Good presentation, data visualization, and critical thinking skills are required to share your perspective with the target audience.
Read more: Top AI chatbot companies in India
What is the Main Difference?
The terms data science and data analytics sound like synonyms to most people, but that is not the case. Data science focuses on understanding the purpose of the dataset and forming the questions the dataset can solve. In contrast, data analytics is a constituent of data science that uses the learning from processed data to answer questions and make decisions. Let us understand the main difference between data science and data analytics:
Data Science vs. Data Analytics: Fundamentals Objective
Data science mainly concerns cross-checking hypotheses, connecting the dots, and forming questions to uncover new patterns that might have gone unnoticed by others. Data scientists collect, process, and explore vast datasets to reach conclusions that solve various problems. They understand data from past, present, and future perspectives via techniques like data mining, analyses, and machine learning. Data scientists can gain significant insights by leveraging unsupervised learning like clustering, deep learning, principal component analysis, neural networks, etc., and supervised learning such as regression, classification, etc. Their main objective is to find a new approach and give a fresh perspective to produce insights from the gathered data.Â
However, data analytics involves answering questions to create profitable business decisions. With the help of existing information, it concentrates on particular areas with a specific objective. It is a more well-defined subpart of data science and focuses mainly on analyzing the data rather than processing or predicting it. Data analytics aims to use big data and find an intelligent solution that can give better results quickly when implemented. It fundamentally converts statistical data into a representable form like charts, tables, and spreadsheets to obtain meaningful insights.
Data Science vs. Data Analytics: Tools used
Handling zettabytes of raw data to discover valuable outcomes requires specific data science tools that have predefined workflows, functions, and algorithms. For example, Statistical Analysis System (SAS) is an advanced analytics tool that deals with various statistical operations such as data mining, econometrics, time series analysis, etc. Distributed process of big data is managed via Apache Hadoop, while TensorFlow for deep learning, machine learning, and artificial intelligence. Even programming languages like Python and R have a comprehensive collection of libraries, such as Seaborn, Numpy, etc., for various phases in the data science lifecycle.
For data analytics, you can use Microsoft Excel, Google Analytics, Power BI, Apache Spark, etc. Excel is one of the most used tools for data analysis to find meaningful insights from the data. The features enable easy real-time collaboration and uploading of data via photos; however, you need to subscribe for advanced features. Tableau, on the other hand, is a free and effective business intelligence tool where you can spend your time on data analysis rather than on data wrangling. Power BI or Google Analytics is a good choice if you have no technical knowledge.
Data Science vs. Data Analytics: Job role
Data science has five main job roles: data scientist, data analyst, data engineer, business intelligence specialist, and data architecture.
A career path in data science has a different education requirement than that for data analytics. Most data analytics jobs do not require a degree in data analysis; a bachelor’s in a similar field is valid; however, you would need more advanced college degrees or specializations in data science with data science courses.
A computer science or mathematics major is preferable for data science jobs. On the other hand, if you have a degree in a related field and want to switch to a career in data science, then data analytics is a good stepping stone. While switching fields, working on personal projects, or getting certification is a great way to display domain knowledge.
The Data Scientist job profile consists of processing, cleaning, and verifying data integrity using machine learning workflows. They should understand artificial intelligence, cloud platforms, and data science concepts. By combining computer science, statistics, mathematics, and modeling skills, data scientists design and build workflows for analyzing the data.
Read more: Free Data Science Courses
The job role of a data analyst involves exploratory data analysis, data cleaning, finding new patterns, and developing easy-to-understand visualizations. A data analyst identifies, collects, cleans, researches, and interprets business data to produce essential insights to make better business judgments. Besides dealing with data, they must collaborate with business leaders, understand the problem, and provide effective solutions. Data analysts should recommend new techniques and strategies to enhance the marking campaigns. They need to ensure that KPIs are reviewed and published regularly. Another critical task is keeping track of the company’s performance and finding improvement areas.
What Should you Choose?
Before jumping into which path is more suited for you, let us reflect on the difference between the two. Data science mainly deals with data collection, storage, and optimization. Advance technical aspects such as machine learning, deep learning, neural networks, and statistics are involved in this field. Data science is a broad subject where data analytics is a part of the data science domain. Data analytics answers questions by analyzing and finding insights from existing data.
Now that you have understood the difference between data science and data analytics, you must be confused about the right career path. You can decide which course is more fitting depending on your interests and skills. For instance, if you want a more technical and mathematical inclined role, then data science is a good choice. While you are creative, a problem solver, and fond of discovering new insights from data, you will enjoy working as a data analyst. If your goal is to become a data scientist, start in the data analytics domain and work your way up or take professional courses to get the required qualification.
Conclusion
There is a fine distinction between data science and data analytics, even though both deal with data. Data science is a broad domain that deals with various processes regarding data, from collecting to storing and filtering it to finding a purpose. Meanwhile, data analytics identifies problem areas and improves them via insights derived from already present data. Data science and data analytics are both in demand nowadays; Therefore, do proper research, understand your interests and work on enhancing your skills to land a job in these fields.