OpenAI has released Whisper, an open-source automatic speech recognition system that the company says allows for “robust” transcription in various languages as well as translation from those languages into English.
Due to an increasing use of smartphones and voice assistant devices, multilingual speech recognition is the need of the hour. The demand for multilingual automatic speech recognition that can handle linguistic and dialectal differences in languages is growing as globalization progresses. While most speech recognition tools cater to English-speaking users, English is not the most spoken language in the world. This implies that the lack of language coverage can create a barrier to adoption.
In addition, the use of more than one language in conversation is a typical occurrence in a culture where individuals are bilingual or trilingual, which makes the development of multilingual models a reasonable case. It is also quite possible that most of the languages in a multilingual setting can have the same cultural heritage resulting in identical phonetic and semantic characteristics. Moreover, the absence of a well-known multilingual voice recognition system draws attention to an exceedingly fascinating field of speech recognition research that monolingual systems have long dominated.
The researchers trained Whisper using 680,000 hours of multilingual and multitask supervised data acquired from the web. According to OpenAI’s blog post, using such a large and diverse dataset improves the system’s ability to adapt to accents, background noise, and technical language.
While the variance in audio quality can aid in the robust training of a model, variability in transcript quality is not as advantageous. Initial examination of the raw information revealed a large number of substandard transcripts. This is why OpenAI created several automatic filtering techniques to enhance the quality of transcripts. The company also noted that many online transcripts were produced by other automatic speech recognition systems rather than by actual humans. A recent study has demonstrated that training on datasets containing both human- and machine-generated data can considerably harm translation system performance. Therefore, OpenAI developed numerous heuristics to find and exclude machine-generated transcripts from the training dataset to prevent the system from picking up “transcript-ese.”
In order to validate that the spoken language matches the language of the text according to CLD2, OpenAI additionally employed an audio language detector that was developed by refining a prototype model trained on a prototype version of the dataset on VoxLingua107. The research team excluded the (audio, transcript) combination as a speech recognition training example from the dataset if the two do not match.
Read More: OpenAI’s DALL-E now offers Outpainting Feature to Extend Existing Images and Artworks
OpenAI selected an encoder-decoder Transformer for the Whisper model’s architecture because it has been proven to scale efficiently. An 80-channel log-magnitude Mel spectrogram representation is generated on 25-millisecond windows with a stride of 10 milliseconds after all input audio was divided into 30-second chunks and re-sampled to 16,000 Hz. This input representation is processed by the encoder using a ‘small stem’ composed of two convolution layers with a filter width of 3 and the GELU activation function. The encoder Transformer blocks are then applied to the output of the stem after adding sinusoidal position embeddings.
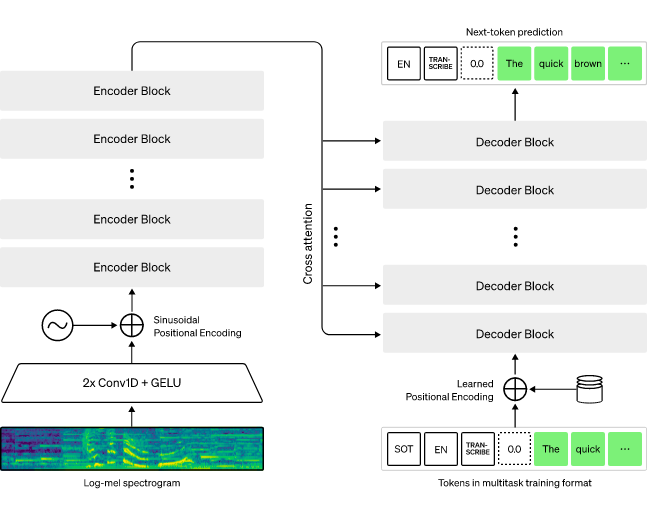
The encoder output is subjected to a final layer normalization just after the transformer employs pre-activation residual blocks. The decoder predicts the corresponding text caption using learned position embeddings, tied input-output token representations, and unique tokens that instruct the single model to carry out various tasks. These tasks include language identification, phrase-level timestamping, multilingual speech transcription, and to-English speech translation. The width and quantity of transformer blocks are the same for the encoder and decoder.
Whisper aims to provide an integrated, resilient speech processing system that operates consistently without requiring dataset-specific fine-tuning to get high-quality results on particular distributions. Whisper’s capacity to generalize effectively across domains, tasks, and languages was examined by OpenAI using an extensive collection of existing speech processing datasets. Rather than using the conventional evaluation protocol for these datasets, which includes both a train and a test split, the researchers tested Whisper’s zero-shot performance and discovered that it is far more resilient and has an average relative error reduction of 55% fewer errors when evaluated on other speech recognition datasets. It outperforms the supervised SOTA on CoVoST2 to English translation zero-shot. Whisper can transcribe speech with 50% fewer mistakes than prior models. However, it does not outperform models specializing in LibriSpeech performance, a competitive benchmark in speech recognition, because it was trained on a broad and diverse dataset rather than being tailored to any particular one.
OpenAI claims that about a third of Whisper’s dataset is non-English. Even though this is an impressive feat, there is a strong possibility of a data imbalance if the training data have different quantities of transcribed data available for each language because of the disproportion in the distribution of speakers in different languages. As a result, languages over-represented in the training dataset could have a greater effect on a multilingual automated speech recognition system. Given that the majority of languages have less than 1000 hours of training data, OpenAI hopes to make an intensive effort to increase the amount of data for these more uncommon languages to bring significant improvement in the average speech recognition performance with only a modest increase in the size of the training dataset.
The OpenAI team speculates that optimizing Whisper models for decoding performance more directly via reinforcement learning and fine-tuning them on a high-quality supervised dataset could also minimize long-form transcribing errors. The researchers only examined Whisper’s zero-shot transfer performance in this research since they were more interested in the resilience characteristics of speech processing systems. Although this environment is essential for research since it reflects overall dependability, OpenAI believes it is possible that findings can be tweaked further for many areas where high-quality supervised speech data are provided.
For now, Whisper’s multilingual capabilities would be a huge asset in the fields of international trade, healthcare, education, and diplomacy. The company decided to release Whisper and the inference code as open-source software so that they could be used to develop practical applications and to do more research on effective speech processing.