A team of engineers at Optics Laboratory of EPFL (Swiss Federal Institute of Technology Lausanne), in collaboration with the Laboratory of Applied Photonic Devices, created a machine learning approach called SOLO, for Scalable Optical Learning Operator, that can detect and categorize information structured as two-dimensional pictures. Their research was just published in the journal Nature Computational Science.
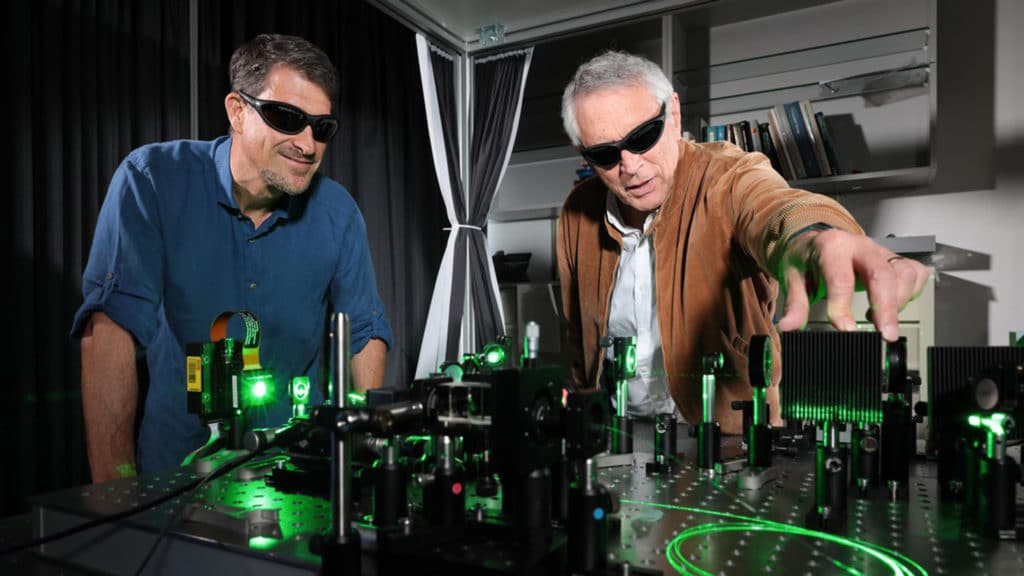
Computers have leveraged deep learning to identify objects in images, transcribe speech, translate between languages, diagnose medical conditions, drive cars, and more. Deep learning is a form of machine learning that is inspired by the human brain’s structure. Through the use of a multi-layered structure of algorithms known as neural networks, deep learning seeks to come to the same conclusions that humans would. But for the training of deep neural networks, huge datasets are required both in terms of quality and quantity. And processing the data to make it optimized for AI training is no less than a scientific feat itself. These, however, come at a significant cost in terms of computer resources and energy consumption. It is no surprise that engineers and computer scientists are working feverishly to find better techniques to train and run deep neural networks.
Current data center networks are witnessing an exponential rise in network traffic as a result of this massive data expansion. With the fast growth of server-to-server communication, meeting the expanding data storage and processing requirements with present technology has become a challenge.
To assuage this crisis, optical networks, which employ light-encoded signals to transfer data in many sorts of networks, pose as a viable alternative.
A neural network can be thought of as a collection of interconnected nodes where each node acts as a neuron of a human brain. However, in a neural network, each neuron has a numeric value and the connection between two neurons is represented by another numeric value called weights. The strength of the neural connection and weights change on the basis of what a neural network learns by training.
To accomplish a task, say image classification, scientists seek a set of weights that will allow the neural network to do so. Each task and dataset have a unique set of weights. The values of these weights cannot be predicted in advance, therefore the neural network must learn them. Each neuron’s state is determined by the weighted sum of its inputs, which is then subjected to a nonlinear function known as an activation function. This neuron’s output subsequently serves as an input to a variety of other neurons.
In June 2019, the University of Massachusetts at Amherst published a shocking paper on the consumption of energy by neural networks. The study reveals that the amount of electricity required for training and searching a BERT (Bidirectional Encoder Representations from Transformers) neural network architecture results in the production of around 626,155 tons of carbon dioxide. This means that the neural network has a carbon footprint of 284 tons, which is five times that of an ordinary car’s lifetime emissions.
Optical computing has promised better performance while using far less energy than traditional electronic computers for a long time. However, scientists have struggled to create the light-based components required to outperform conventional computers, putting the idea of a viable optical computer in jeopardy. Amid these difficulties, the SOLO team’s research has promised new insights.
The objective of the study was to use different processing methods, particularly photonics, to minimize the energy requirement. As a result, the team considered utilizing optical fibers to do some calculations, which were carried out automatically by light pulses propagating inside the fiber. This simplifies the computer’s architecture, retaining only a single neuronal layer, making it a hybrid system,” says Ugur Tegin, the lead co-author of the study.
Read More: Optical Chips Paves The Way For Faster Machine Learning
A feasible optical computer (including a neural one) must include linear and nonlinear parts and input-output interfaces while maintaining the speed and power efficiency of optical interconnections, according to the paper.
As a result, the engineers devised SOLO, which is a combination of the linear and nonlinear components of an optical system in a shared volume confined in multimode fiber (MMF).
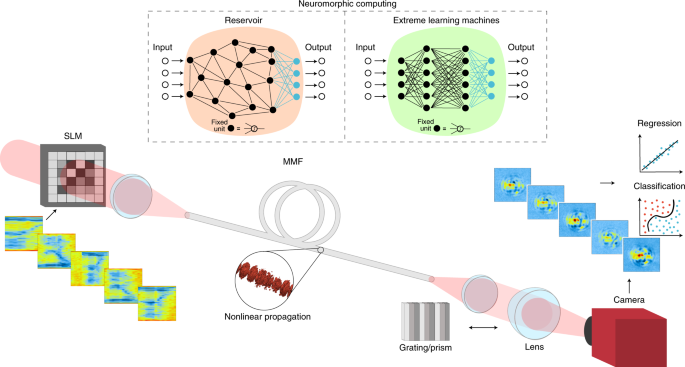
The data to be processed is entered into the left-hand two-dimensional SLM (spatial light modulators). The light from a pulsed light source is nonlinearly transformed as it propagates through the fiber at sufficiently high illumination peak power, and the output of the computation is projected on the two-dimensional camera. The MMF’s input-output operation is fixed and extremely nonlinear due to the qualities of the fiber and laser source. On the basis of a huge dataset of input-output pairs, the team combines a fixed nonlinear MMF mapping in the optical domain with a single-layer digital neural network (decision layer) that can detect output captured on the camera.
Engineers used a collection of X-ray pictures of lungs afflicted by various illnesses, including COVID-19, to test this novel technique. They used SOLO to identify the coronavirus-affected organs in the data. To compare the data, they put it through a standard neural network system with 25 layers of neurons.
While the X-rays were classified equally effectively by both methods, SOLO used 100 times less energy. This was the first time the team showcased quantifiable energy savings. They believe that SOLO’s improved energy efficiency may potentially offer up new possibilities in other fields of ultra-fast optical computing.