When navigating in a new environment, humans generally rely on visual, spatial and semantic cues that can assist them in getting to their destination quickly. Suppose you are invited as a guest to a new house of your friend, you can make sensible predictions about the placement of goods in their respective rooms or depend on visual cues to locate the living room in the house.
This may be quite challenging for robotic agents trying to perform similar navigation around a given space.
The most common method leveraged is model-free reinforcement learning to learn implicitly what these cues are and how to apply them for navigation tasks in an end-to-end approach. However the problem with this is that, navigation cues learned via reinforcement learning are too costly, difficult to examine, and impossible to re-use in another agent without starting again.
A world model, which encapsulates rich and relevant information about their surroundings and allows an agent to make explicit predictions about actionable events within their environment, is an intriguing alternative for robotic navigation and planning agents. With outstanding results, such models have sparked significant interest in robotics, simulation, and reinforcement learning, including the discovery of the first known solution for a simulated 2D automobile racing problem and human-level performance in Atari games. However, when compared to the complexity and diversity of real-world landscapes, gaming worlds are still very simple.
Pathdreamer is a novel world model announced recently by Google AI that creates high-resolution 360 degrees visual views of regions of a building unseen by an agent, using just limited seed observations and a suggested navigation route. By constructing an immersive scene from a single point of view, Pathdreamer predicts what an agent would see if it moved to a new point of view or even to an area that had previously been unseen, such as around a corner. This approach can also aid autonomous entities in navigating the real world by encoding information about human environments.
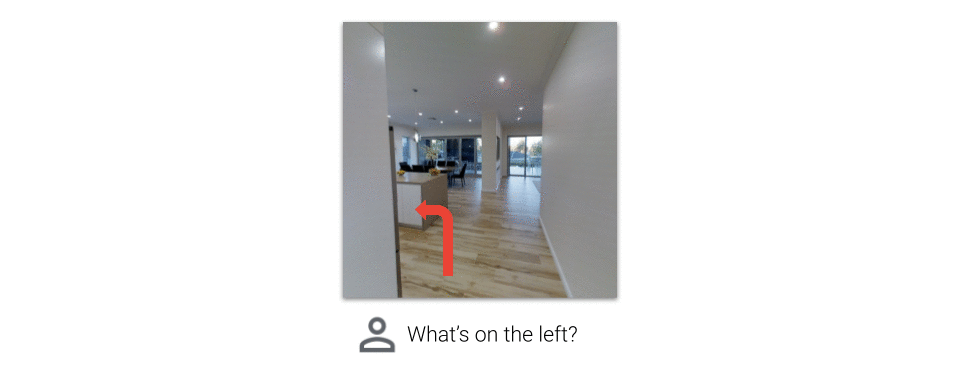
By increasing the amount of training data for agents, these world models can help in training agents in the model.
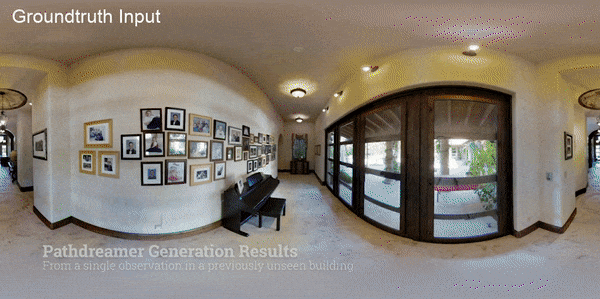

Pathdreamer takes a series of previous observations as input and provides predictions for a trajectory of future locations, which the agent engaging with the returned observations can supply either upfront or iteratively. Both the inputs and the predictions employ RGB, semantic segmentation, and depth images. Pathdreamer internally uses a 3D point cloud to represent surfaces in the world. Each point in the cloud is labelled with the RGB colour value as well as the semantic segmentation class, such as wall, chair, or table.
The point cloud is initially re-projected into 2D in the new site to give ‘guidance’ pictures for predicting visual observations in a new location. Next, Pathdreamer uses these photos to produce realistic high-resolution RGB, semantic segmentation, and depth. New observations (actual or projected) are gathered in the point cloud as the model ‘moves.’ The use of a point cloud for memory has the advantage of temporal consistency: revisiting locations are displayed in the same way as earlier observations.
Converting guiding pictures into convincing, realistic results entails two distinct stages by Pathdreamer — both of which are powered by convolutional neural networks. The structure generator (a stochastic encoder-decoder) generates segmentation and depth pictures in the first step, and the image generator (image-to-image translation GAN) converts them into RGB outputs in the second stage. The first step construes a feasible high-level semantic description of the scene, which is then rendered into a realistic color image by the second stage.
The Google AI team used Matterport 3D RGB panoramas as training targets with a resolution of 1024×512 pixels, and the Habitat simulator to produce ground-truth depth and semantic training inputs and assemble them into equirectangular panoramas for the Image Generator. Due to the restricted amount of panoramas available, the team attempted data augmentation by randomly cutting and horizontally rolling the RGB panoramas. They also employed Habitat to produce depth and semantic pictures to train the Structure Generator. They accomplished data augmentation by perturbing the viewpoint coordinates with a random Gaussian noise vector, since this stage does not require aligned RGB pictures for training.
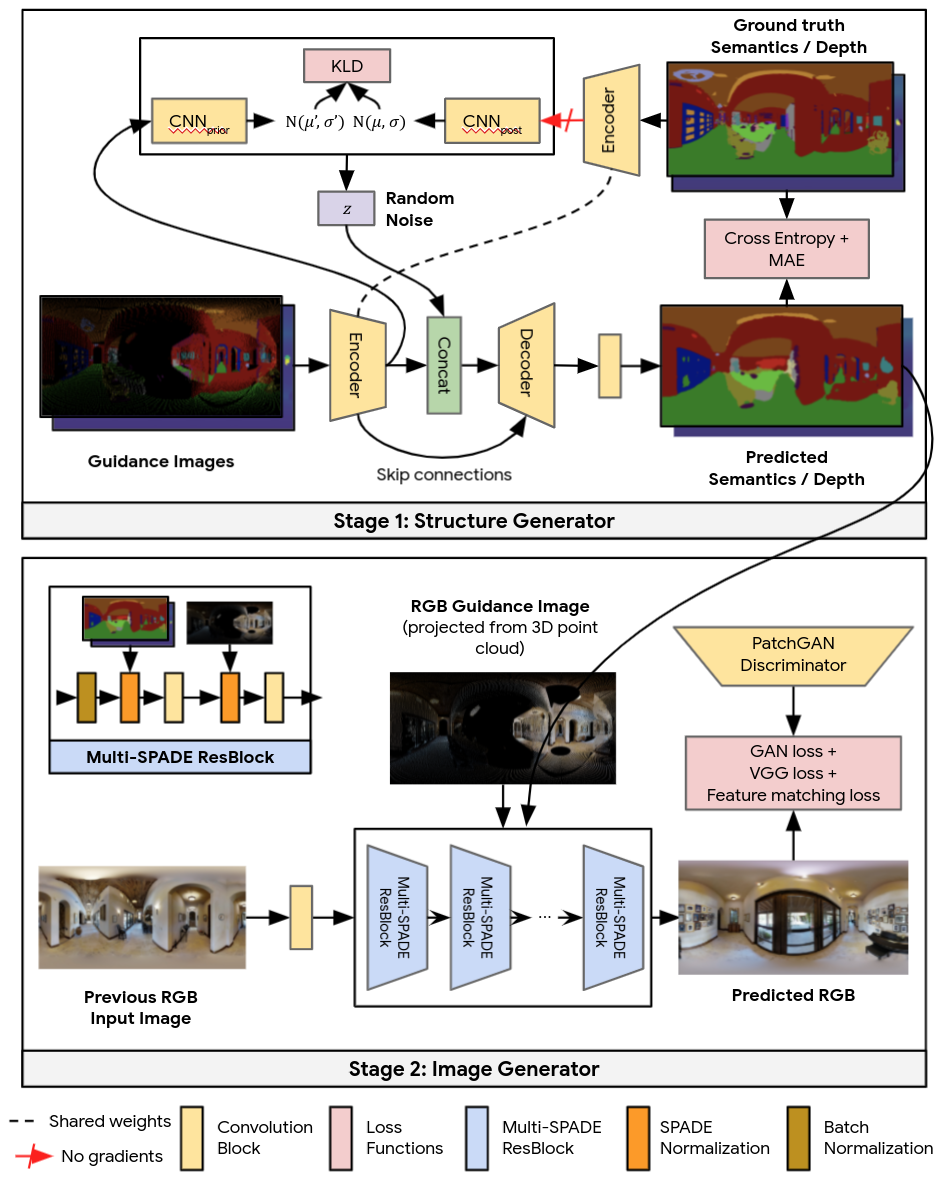
In regions of great uncertainty, such as a location presumed to be around the corner or in an undiscovered chamber, a variety of situations are available. Pathdreamer’s structure generator is conditioned on a noise variable, which represents the stochastic information about the next location that is not recorded in the guiding pictures, using concepts from stochastic video generation. Pathdreamer can create various sceneries by sampling numerous noise factors, allowing an agent to test several conceivable outcomes for a given route. Not only in the first stage outputs (semantic segmentation and depth pictures), but also in the produced RGB images, these different outputs are represented.
Read More: Google TensorFlow Similarity: What’s New about this Python Library?
Finally, the Google AI team tested if Pathdreamer predictions could help with a downstream visual navigation task. Using the R2R dataset, they focused on Vision-and-Language Navigation (VLN) because achieving the navigation goal necessitates properly anchoring natural language commands to visual data, making task-based prediction quality assessment tricky.
The main characteristic of R2R (Room to Room) dataset is that here the agent prepares ahead by simulating various alternative passable paths through a given environment and ranks each against the navigation instructions provided to find the optimal path.
The researchers examined the following three situations as part of the experiment:
- Ground-Truth setting in which the agent plans by interacting with the real world, i.e. through moving.
- The baseline setting where the agent prepares forward but does not move. Instead, it interacts with a navigation graph, which records the building’s accessible paths but provides no visual observations.
- Pathdreamer setting in which the agent interacts with the navigation graph and receives suitable visual observations produced by Pathdreamer to plan ahead without moving.
According to the Google AI researchers, when the agent prepares ahead for three steps in the Pathdreamer mode, it has a 50.4 percent navigation success rate. This result is much higher than the Baseline setting’s 40.6 percent success rate excluding Pathdreamer. This indicates that Pathdreamer stores visual, spatial, and semantic information about real-world interior locations in a meaningful and accessible manner. In the Ground-Truth setting, the agent has a 59 percent success rate.
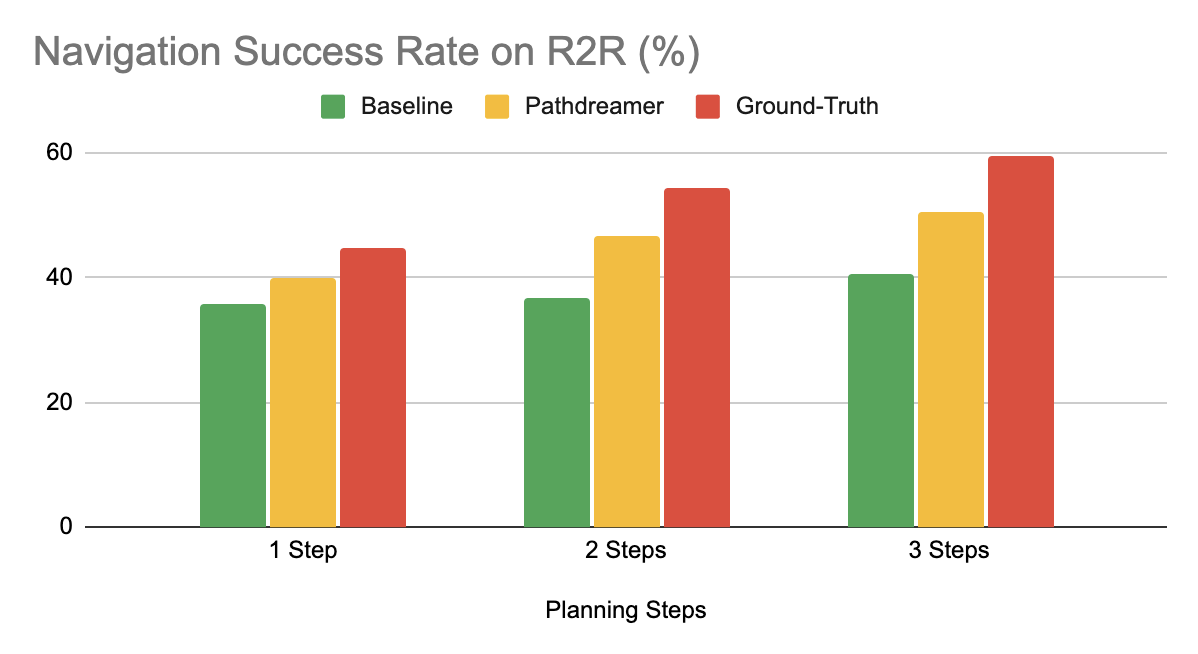
The team, however, points out that in this case, the agent needs to invest substantial time and resources to physically explore a huge number of paths, which would be prohibitively expensive in a real-world scenario.
At present the team envisions Pathdreamer will be used for more embodied navigation tasks, such as Object-Nav, continuous VLN, and street-level navigation.
Read more here.