A new class of materials has been developed by engineers at UCLA in California that can adjust in real-time to dynamic external forces by learning behaviors through time and creating its own “muscle memory.” Known as mechanical neural networks (MNNs), the materials consist of a structural system made up of adjustable beams that may change the shape and behaviors of the material in response to shifting stimuli.
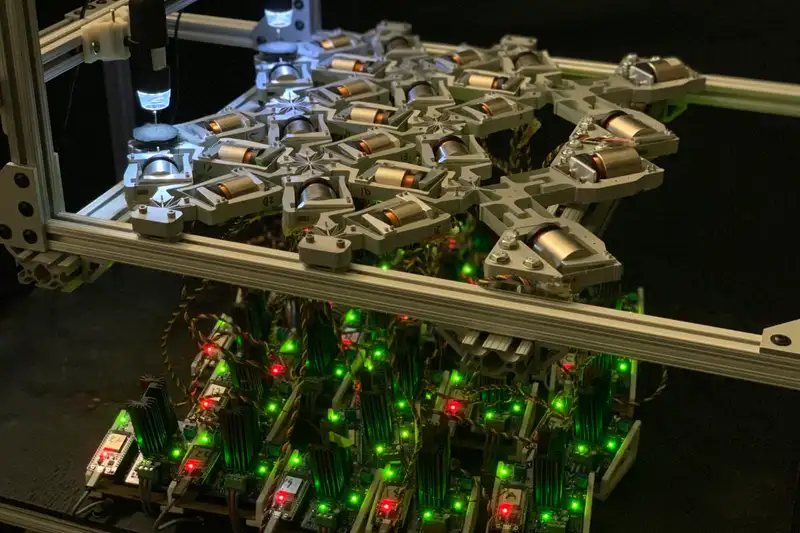
Lee et al., Sci. Robot.
The study’s findings, which have potential in building construction, aviation, and imaging technology, were published in Science Robotics on Wednesday. According to the authors, the experimental study establishes the groundwork for AI-architected materials that can be used in the construction of buildings, aircraft, and imaging technologies.
It was in 1944 when Warren McCullough and Walter Pitts, two University of Chicago scholars who later transferred to MIT in 1952, made the first theoretical proposal for neural networks. A neural network is made up of hundreds or even millions of intricately intertwined simple processing nodes that are vaguely modeled after the human brain. The majority of neural networks used today are composed of node layers that include an input layer, one or more hidden layers, and an output layer.
The authors explained that mechanical neural networks are lattices of linked, tunable beams that unite at nodes and are propelled by input and output forces or displacements. To train the lattice so that it can learn desired mechanical behaviors (such as shape morphing, acoustic wave propagation, and mechanical computation) and bulk properties (such as Poisson’s ratio, shear, and Young’s modulus, and density), the stiffness values of the interconnected beams are optimized as network weights. This is how the new class of architected materials—also known as mechanical metamaterials—got introduced in this research. These materials learn after being exposed to unexpected ambient stress conditions over time.
Prior to this research, acoustic metamaterials, such as the acoustic analog computing (AAC) system, have been proposed by others, but since they are not neural networks, they cannot learn. In 2019, Tyler Hughes et al. suggested an acoustic metamaterial that mimics the behavior of a trained neural network. However, a fabricated version of the proposed design was unable to learn new behaviors since training is done during the design process by simulating the adjustment of the mass within a vibrating plate. While alternative mechanical concepts have also been suggested and tested over the last two years using just simulation, this is the first time the mechanical neural network concept mentioned in this UCLA study has been physically and experimentally verified.Â
Read More: How Google’s GraphWorld solves Bottlenecks in Graph Neural Network Benchmarking?
The concept can also be expanded to complicated three-dimensional (3D) lattices that can fill arbitrary-shaped volumes and meet required fixturing requirements for practical material applications. Further, mechanical neural networks function as deep neural networks that may learn several complicated behaviors at once. This is because they generally have several layers of nodes comparable to the neurons in artificial neural networks. One major advantage of the mechanical neural network is that it has the ability to relearn previously mastered behaviors and learn new behaviors as needed with exposure to changing environmental scenarios, in case it is broken, chopped to occupy an alternative volume, or fixtured differently. This feature is not present in other neural networks.
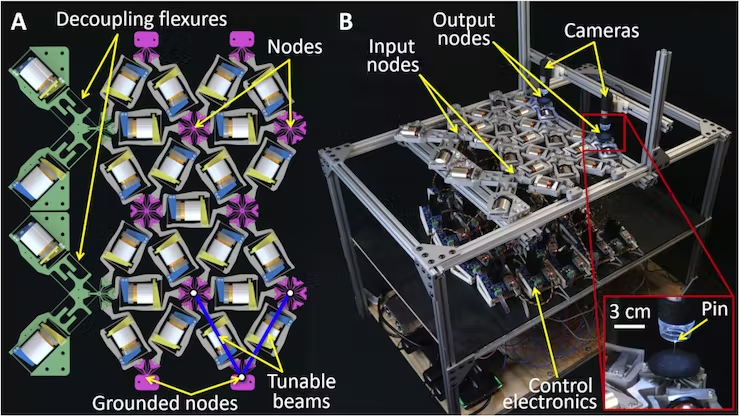
The researchers present an illustration of how the metamaterial might be applied to airplane wings. To increase efficiency and maneuverability, the mechanical neural networks may learn to change the form of the wings in response to changing wind patterns while the aircraft is in flight or incurs internal damage. A mechanical neural network-based wing might stiffen and relax its connections in response to each of these situations to maintain desirable properties like directional strength. The wing gradually adopts and maintains new qualities by iterative algorithmic changes, adding each new behavior to the rest of its repertoire in a manner akin to muscle memory. In applications involving infrastructure, where earthquakes or other natural or man-made disasters pose a concern, study authors pointed out that it is also possible that the material might aid increase stiffness and general stability.
Ryan Lee of the University of California, Los Angeles, and his colleagues created a network of 21 beams, each 15 cm long, and placed them in a triangular lattice. Each beam is equipped with a voice coil, flexures, and strain gauges. According to the researchers, these characteristics allow the beam to modify its length, adapt to its changing surroundings in real-time, and interact with other beams in the system. When forces are applied to the beam, the voice coil is employed to start a precisely tuned compression or expansion. The algorithm controls the learning behavior using information gathered by the strain gauge from the beam’s velocity. The system’s movable beams are connected to it via the flexures.
Every beam has sensors that determine how far each “neuron,” or beam joint, is out of alignment, as well as a tiny linear motor that can change the stiffness of the beam. By adjusting the beam stiffness, a computer can train the network as a result. After this is completed, the structure no longer has to be calculated externally, and the different beam stiffnesses are fixed.
The network’s response to applied forces is controlled by an optimization algorithm, which uses data from all of the strain gauges to create a combination of rigidity values. In order to verify the actions done by the strain gauge-monitored system, cameras were trained on the system’s output nodes. Although early versions had problems with the delay between input and reaction, the team worked for five years to work out the issues until the mechanical neural network material could learn and react in real-time.
Read More: Introducing Autocast: Dataset to Enable Forecasting of Real-World Events via Neural Networks
Even though the system is around the size of a microwave oven, the researchers want to make the mechanical neural network design simpler so that thousands of networks could be produced on the micro-scale within 3D lattices for useful material applications.
The researchers propose that mechanical neural networks could be included in armor to deflect shockwaves or in acoustic imaging technologies to harness soundwaves in addition to being used in cars and building materials.
Where shoud I purchase the mechanical neural network parts. I need them for my research work.