Almost two years ago, American semiconductor company Cerebras challenged Moore’s Law with the Cerebras Wafer Scale Engine (WSE). And now it has unveiled the world’s first multi-million core AI cluster architecture. On its second-generation platform, the new Cerebras CS-2 Wafer Scale Engine 2 processor is also the world’s largest chip – which happens to be a “brain-scale” microprocessor that can power AI models with over 120 trillion parameters.
A chip starts as a cylindrical ingot of crystallized silicon that is roughly a foot in diameter and is sliced into circular wafers that are a fraction of a millimeter thick. A method known as photolithography is then used to “print” circuits onto the wafer. Chemicals that are sensitive to ultraviolet light are meticulously layered on the surface; UV beams are then projected through intricate stencils called reticles, causing the chemicals to react and form circuits.
Traditionally, a 12-inch (30 cm) silicon disc called a wafer is used to produce hundreds or even thousands of computer chips, which are then split up into individual chips. Cerebras, on the other hand, utilizes the complete wafer.

The Cerebras CS-2 chip is designed for supercomputing activities, and it is the second time Cerebras, based in Los Altos, California, has presented a chip that is essentially a complete wafer since 2019. A single WSE-2 chip has the size of a wafer, measuring 21cm across, and contains 2.6 trillion transistors and 850,000 AI-optimized cores — all packed on a single wafer-sized 7nm processor. The largest GPU, by comparison, is less than 3cm across and contains only 54 billion transistors and 123x fewer cores. Cerebras’ latest interconnect technology allows it to chain together numerous CS-2 systems (machines powered by WSE-2) to support much larger AI networks, several times the size of the brain.
Cerebras has announced technology that permits the construction of very large clusters of CS-2s, up to 192 CS-2s, in addition to boosting parameter capacity. Considering that each CS-2 has 850,000 cores, 192 CS-2 clusters would be equivalent to 163 million cores in terms of core count. The Cerebras CS-2 chip is one-third of a conventional rack, requires roughly 20 kilowatts, has a closed-loop liquid cooling system, and needs quite large cooling fans like its predecessor.
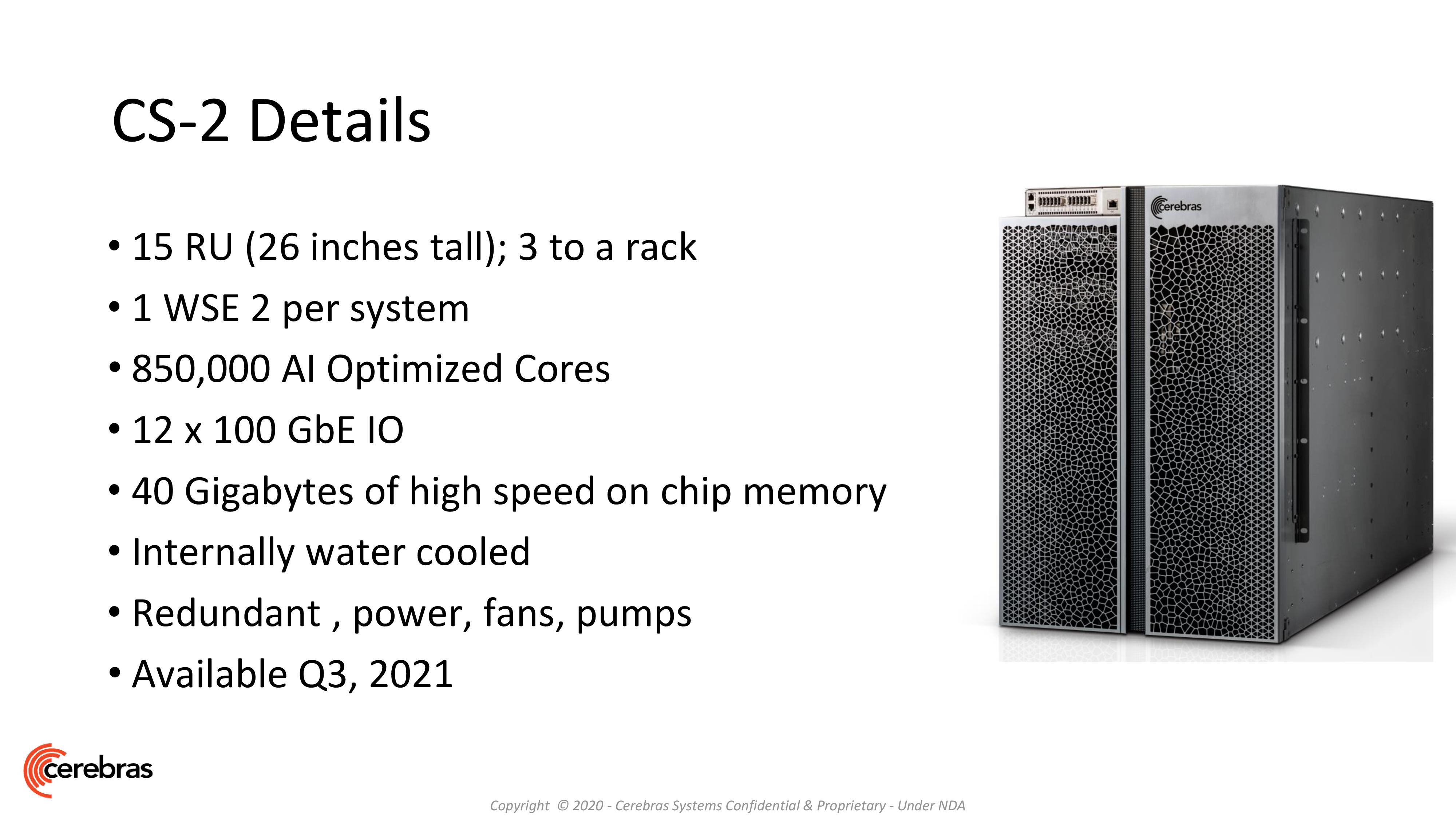
Most AI algorithms are now trained on GPUs, a type of processor that was originally built for generating computer graphics and playing video games but is now well suited for the simultaneous processing required by neural networks. As neural networks are too big for any single chip to hold, most of the large AI models are split amongst dozens or hundreds of GPUs, which are connected by high-speed wiring.
However, one of the most significant constraints was data transfer between the processor and external DRAM memory, which consumed time and energy – making it more tedious when dealing with vast sets of data. The original Wafer Scale Engine’s creators reasoned that the solution was to make the chip large enough to carry all of the data it required with its AI processing cores.
Cerebras has incorporated four new innovative technologies on its new world’s largest chip:
- Cerebras MemoryX,
- Cerebras SwarmX,
- Cerebras Weight Streaming,
- Selectable Sparsity.
Weight Streaming is a new software execution architecture that keeps model parameters off-chip while yet maintaining on-chip training and inference performance. This new execution architecture separates compute and parameter storage, allowing for unprecedented flexibility and independent scaling of model size and training performance. It also solves the problems of latency and memory bandwidth that plague big clusters of small CPUs. This dramatically simplifies the workload distribution model, allowing users to scale from using 1 to up to 192 CS-2s with no software changes.
These parameters are saved in the external MemoryX cabinet, which expands the 40GB on-chip SRAM memory with up to 2.4PB of extra memory, making it act like it is on-chip. The additional memory can help companies run larger brain-scale AI models. MemoryX also offers the ability to process weight updates via internal computation. This is accomplished by streaming the weights onto the CS-2 systems, one layer at a time, to calculate each layer of the network. The gradients are sent in the opposite way back to the MemoryX on the backward pass, where the weight update is done in time to be used for the next training iteration.
Communication across many chips can be difficult using standard approaches that split the whole workload amongst processors, with 20 petabytes of memory capacity and 220 petabits of aggregate fabric bandwidth. Furthermore, because of the chip’s 15kW power consumption rate, scaling the system’s performance across multiple systems is extremely difficult. This necessitates specialized cooling and power supply, making cramming more wafer-sized processors onto a single system almost impossible.
Cerebras leverages the new SwarmX fabric technology, which enables multi-system scalability, to address these challenges. This is an AI-optimized communication fabric that uses Ethernet at the physical layer but employs a customized protocol to transport compressed and reduced data across the fabric. SwarmX effectively transforms the CS-2 hardware into a black box to which weights and gradients are delivered and processed. Since each CS-2 has 850,000 cores and 40 GB of on-die memory to execute the whole model, there is no requirement for model parallelism in this method.
Overall, SwarmX allows CS-2s clusters in achieving near-linear performance scaling and can connect up to 163 million AI-optimized cores across up to 192 CS-2s.
Cerebras is lowering computational complexity by exhibiting up to 90% sparsity with almost linear advantages of speedup utilizing Selectable Sparsity, whereas most AI processors can only manage 50% sparsity. Selectable Sparsity allows users to choose how much weight sparsity they want in their model, resulting in a direct reduction in FLOPs and time to solution. It aids CS-2 in producing faster responses by reducing the amount of computing work necessary to arrive at solutions.
Read More: Baidu Announces Mass Production of Kunlun II AI Chip at its Annual Event
Unlike prominent names like NVIDIA, Cerebras is beating its own drums by innovating against physical realities and economic limitations of Moore’s law and the AI chip industry. Using Cerebras CS-2 chip, the company aims to go beyond the usage of chips for neural network training to performing massive parallel mathematical operations. Some of its customers are Argonne National Labs, Lawrence Livermore National Lab, Pittsburgh Supercomputer Center, GlaxoSmithKline and AstraZeneca, and “military intelligence” organizations.