Data science is said to be the hottest profession of the 21st century. With the buzz about its integral role in today’s business and research landscape, organizations are rushing to leverage it to achieve their enterprise goals. Companies are swiftly tapping on this emerging technology to harness the power of their data and offer more value to consumers, resulting in an increase in demand for data scientists.
Data science has been rapidly gaining traction in the industry and shows no signs of slowing down due to the development of advanced machine learning algorithms and a massive shift from descriptive to predictive analytics. This is where businesses look for new and experienced talents in the data industry who can come up with new ways to develop and experiment ahead of time.
In an exclusive interview with Dattaraj Rao, Chief Data Scientist of Persistent Systems, we tried to decipher the Data Science Culture at the company.
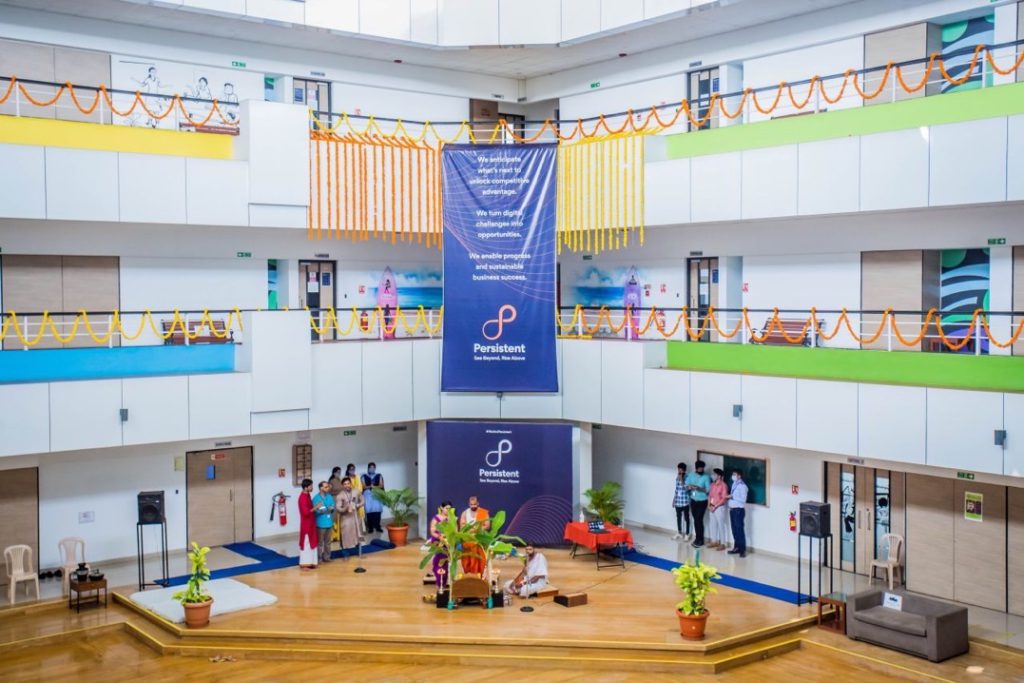
Founded in October 1990, Persistent Systems specializes in software product idea and design, agile product engineering, performance engineering, support assurance, quality assurance, and other technical services. Using an assortment of technologies like big data analytics, cloud, embedded systems, and security, the company has built solutions that center around promoting Responsible AI practices.
Building Trust with Responsible AI
As Responsible AI has become a necessary add-on while developing AI solutions today, companies like Persistent Systems are helping the tech industry get close to making this mainstream. Persistent Systems has a mature data science practice that helps it cater to its customers in Healthcare and BFSI domain through Responsible AI systems and solutions. Its Responsible AI strategy focuses on making AI/ML systems reproducible, transparent, accountable, private, and secure. For this, the company puts emphasis on standardization of the ML pipeline using MLOps patterns like data catalog, model registry, feature store, low-code ML, AutoML, data & concept drift monitoring, and Explainable AI (XAI).
Persistent Systems has benchmarked state-of-the-art Explainable AI libraries to understand model predictions and evaluate inherently interpretable glass-box models. Dattaraj explains ‘Model development may use standard frameworks like PyTorch, TensorFlow, Spark ML or we could leverage no-code/low-code ML tools like H2O.ai and PyCaret or we could use a Cloud stack like Sagemaker or Azure ML Studio.” He adds that while the actual tech stack may vary with the customer, the core patterns remain common and help in customer adoption.
Pre-requisites of A Successful Data Scientist
Dattaraj strongly supports the notion that a data scientist should be able to tell a good story with the data by clearly articulating how a problem is solved and verifying it with appropriate metrics. As someone with an engineering degree, he thinks a solid background in math and statistics is very important to succeed as a data scientist.
He acknowledges that the ability to use a library is impressive, but one needs to know the inner workings of the ML algorithms to be able to apply them correctly and tune models to solve a problem. In addition, a good data scientist should clearly understand the problem domain and the different types of data sources. Dattaraj says, “A significant amount of time is spent in understanding and cleansing the data and more effort you spend upfront will help you build better models.”
Data Science Hiring Practices at Persistent Systems
At Persistent Systems, Dattaraj reveals, there are multiple interview levels with programming exams to interviews on specific math and problem-solving skills. In addition to possessing the right mix of positive attitude and problem-solving skills, the hiring team also looks for candidates having a basic knowledge of a relevant domain. This is a must because, many times, data scientists have to decipher complex data sources and find useful features using the given resources. Therefore, having a knack for learning a new problem domain and being hands-on with data analysis tools can give the candidates an edge over the rest.
Along with the aforementioned, Dattaraj adds that Persistent Systems also values data science certifications. However, the final decisions about hiring a candidate will be primarily based on the skills, values, and problem-solving attitude they bring to the interview. Dattaraj also affirms that the industry experience of a candidate is always preferred since a major part of work for the data scientist is to understand the problem and data sources. Experience with solving a business problem is valuable, and industry experience will definitely be an advantage.
Read More: Data Science and Machine Learning jobs are rising in 2022 says LinkedIn
Acclimating to New Normal Hiring Practices
During the pandemic, Persistent Systems had no hassles in finding some amazing talent, and their hiring record is at an all-time high. According to Dattaraj, the company’s enterprise IT teams have adopted the same standards of excellence that it brought to its customers and faced no problem while transitioning to WFH culture. Persistent Systems adopts the best in class standards for network management and cybersecurity, making it possible for its engineers and data scientists to perform at maximum efficiency. Today, the company has talented minds from all over the world as an integral part of the Persistent family. Together, they help it offer unmatched results and performance that meet customer expectations.
Preparing for Dynamic Industry Demands
Speaking on future career scope, Dattaraj informs that AI/Ml is an extremely fast-evolving space. For better career prospects, candidates need to keep themselves updated on the latest research and upcoming trends. Consequently, Dattaraj encourages readers to catch up on research papers, blogs, conference videos published in their area of interest. The best thing about data science is there is so much material available for free on the internet. For example, there has been an influx of some unique ML models for text analytics in the last couple of years. Most of these are available for free and there are ready-to-run notebooks available at Google Colab, Kaggle, or Github that show example usage. New tools (like MLOps, AutoML, Feature stores)and patterns are continuously making the job of data scientists easier. In retrospect, candidates need to be at least aware of these concepts.
Words of Wisdom
Dattaraj asserts that today there are a lot of opportunities and avenues for someone aspiring to be a data scientist. He insists, “Keep learning and think of how you can make your and others’ jobs easier with the application of AI.”
Dattaraj claims to have witnessed many young data scientists who are very good programmers but don’t take the additional effort to understand ML algorithms and rely too much on the libraries and API calls. “I feel the time spent in understanding the basics behind models is definitely worth the effort and will make you better data scientists,” he concludes.