Recently, researchers from Tohoku University have unraveled why people with autism read facial expressions differently using a neural network model. The results of this study were published in the journal Scientific Reports on July 26, 2021.
In individuals with autism spectrum disorder (ASD), problems with facial emotion recognition (FER) are prevalent. The problem isn’t with how information is encoded in the brain signal, but rather with how it is interpreted. In other words, individuals with autism spectrum disorder understand facial expressions differently than healthy people. As patients get older, the characteristics that identify autism, such as sensory and emotional problems, repetitive behaviors, and a lack of social subtlety, can make it difficult to manage too.
According to Yuta Takahashi, one of the paper’s co-authors, by looking at facial expressions, humans can detect distinct emotions such as sadness and anger. However, little is known about how humans learn to distinguish distinct emotions based on facial expressions’ visual information. Yuta also mentioned that earlier, scientists were not sure what happens in this process that causes individuals with an autism spectrum disorder to have trouble reading facial expressions.
To understand this better, the researchers devised a predictive processing theory. According to this hypothesis, the brain is continuously predicting the next sensory experience and adapting when it is incorrect. Sensory data, such as facial expressions, aid in the reduction of prediction error.
Based on predictive processing theory, the team developed an artificial neural network [hierarchical recurrent neural network] that was able to mimic the developing process. It achieved this by training itself to predict how different regions of the face will move in facial expression videos. The main goal was to use a developmental learning method to train a neural network model to predict the dynamic changes in facial expression movies for six fundamental emotions without explicit emotion labels.
The next stage was to self-organize the emotion clusters into the higher level neuron space of the neural network model. At the same time, the model had no idea what emotion the video’s face expression represented. The neural network model was also able to generalize unknown facial expressions that were not included in the training stage, as well as recreate facial part movements with minimal prediction errors.
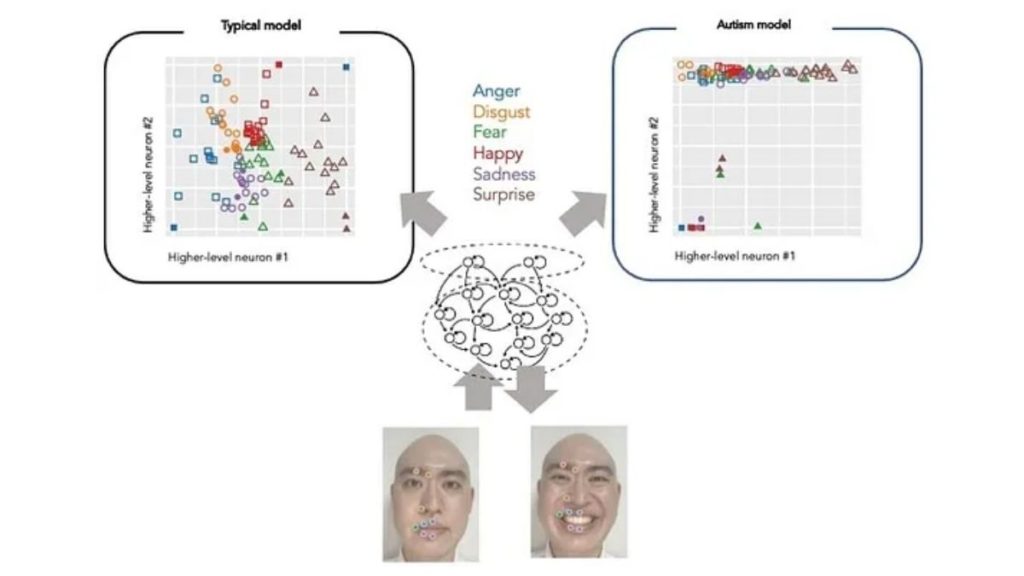
During the tests, the team of researchers introduced anomalies in the neurons’ activity, which provided insight into the influence on learning development and cognitive characteristics. The experiments showed that generalization ability dropped in the neural network model when the heterogeneity activity in the neuronal population was lowered. This showed that the development of emotional clusters in higher-level neurons was suppressed, which resulted in the neural network model failing to detect the emotion of unfamiliar facial expressions, a sign of autism spectrum disorder.
Read More: Latest AI Model From IBM, Uncovers How Parkinson’s Disease Spreads in an Individual
“Using a neural network model, the study demonstrated that predictive processing theory can explain emotion detection from facial expressions,” says Yuta. The findings also support the previous studies that impaired facial emotion recognition in autism spectrum disorder can be explained by altered predictive processing and provide possible insight for investigating the neurophysiological basis of affective contact. This will also help researchers with a better understanding of the neurophysiological foundation of affective contact.
“We hope to further our understanding of the process by which humans learn to recognize emotions and the cognitive characteristics of people with autism spectrum disorder,” added Yuta.