Oorwin is an AI-powered recruiting platform that seeks to enhance hiring quality, increase recruiter efficiency, and boost revenue by transforming traditional recruitment procedures. It offers a fully-integrated ATS, CRM, and HRMS platform to deliver efficiency and growth for staffing and consulting firms. Recently, we had an opportunity to get in touch with Sai Vignan Malyala, Principal Data Scientist at Oorwin, to understand the company’s Data Science Culture.
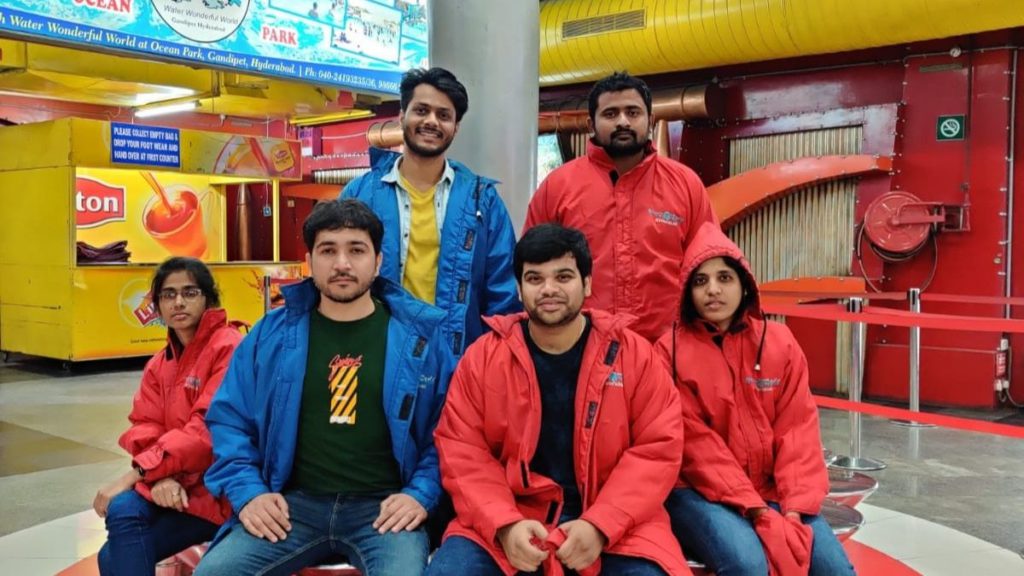
5 Ways Data Science is Leveraged at Oorwin
- To improve user efficiency: Oorwin strives to build features that improve the efficiency of the recruiter/user and close more in less time accurately. In this context, some of its features are resume parsers, job description parsers, other document parsers, recruitment pre-screening chatbots, email classifiers with insights generation, personality insights, job closure probability, salary prediction, etc. All of these are important and make users’ work pretty easy and save their time.
- Recommendation engines to improve users’ choices: Typically, handling a lot of data and making decisions, especially on jobs, can seem daunting for users. For instance, an individual picks out favorite songs and curates a workout playlist. For its business clients, Oorwin has built recommendation systems like semantic search, similar profiles, and similar jobs.
- To grab data and give insights: Oorwin has built more than 60 impeccable scraping engines working all day gleaning different market data for better analysis and insights. This data is used in its model training and the rest to provide market insights necessarily via modeling. Some of these data analysis services include Job Grabber & Insights, Profile Grabber & Insights. At present, Oorwin is working on a recruitment analytics solution that will help users make the right decisions at the right time as per their data and business actions.
- Business Analytics to make data-driven decisions: Companies may build plenty of data science use cases with a lot of effort, but it would be worth only if users benefit. That’s why most of the companies have only a 2% success rate on their features. Sai Vignan believes the ‘wow factor is not the key, but the need of the feature.’ Consequently, Oorwin always ensures to analyze its feature’s usage and trends. Sai reveals, “We have a good cluster set for this. Fortunately, we really get great trends insightful enough to choose our next feature to build or enhance. We always have a team doing continuous POC’s checking the need and possibility of different use-cases.”
- Data Pipelines & Engineering: The data science team at Oorwin is well equipped with Data Engineering skills to set up data pipelines for training models and deploy those models in production using variant methods as per model.
Fostering Innovation with Disruptive Technologies
As consumer behavior has changed since last year, companies had to introduce new additions in their tech pipeline to cater to the new normal of client expectations. Since last year Oorwin has taken drastic measures to boost collaboration with its clients. For instance, the product usage trends and analysis showcased in dashboards have helped Oorwin identify better applications and use-cases of their solutions during the pandemic. Employees at Oorwin tried to understand what was needed in the product for users during the pandemic. They observed that healthcare-related recruitment had grown more than 40% during the pandemic. This observation inspired the company to improve its models in that domain. Some of the tools used by Oorwin include Python, Streamlit, Power BI, PyTorch, spaCy, hugging face transformers, Gensim, FastAPI, AWS for Dockerization, AWS Lambda, AWS Metrics, and more. Besides, they use custom tools for tagging, training and monitoring data pipelines.
Cultivating the Right Mix of Data Science skills
“To become a successful data scientist, one has to become a good data analyst first. Unless one understands what data he has and what data he wants as per domain knowledge, he cannot build anything meaningful,” exclaims Sai Vignan.
From a generic viewpoint, analytical mindset, ability to build the problem statement, eagerness to learn the data with the right attitude, strong communication skills (to integrate or collaborate with other teams on the use cases) are the primary skills for any successful data scientist.
From a technical viewpoint strong foundation in statistics, algorithms, programming, and domain expertise is a must for successful data scientists.
According to Sai Vignan, there isn’t any ready-made approach to build something in data science. It’s all about the ability to draw insights and create something new from the data. The work of a data scientist comprises both tedious data cleaning and interesting model-building. Therefore, the right mindset is required to excel in this profession.
Data Science Hiring Process of Oorwin
Oorwin ideally looks for people with a strong foundation in programming, statistics, and basic ML/DL/NLP algorithms. However, getting the right candidate is always a concern; thus, the company has a standardized approach of pre-screening and interviewing. In other words, Oorwin has unique criteria of post-selection screening for the right attitude in the candidate suitable for the company. Recruiters favor candidates possessing a blend of skills like humility, dedication, eagerness to learn, and a collaborative demeanor.
Oorwin also boasts of having candidates who are freshers in its workforce. Sai Vignan explains that the eagerness to learn and grow with humility in freshers is unparalleled compared to candidates having industry experience. Oorwin still follows that culture of hiring some freshers, training them internally or externally, and offering them opportunities to develop impressive solutions for the company. Though initially, it will be pretty challenging to build accurate models. Sai Vignan advises data scientists not to get discouraged but to keep the determination to learn from mistakes and make better models in the future. The company also values data science certification during hiring. However, evaluation is purely based on skills and what candidates learned in certification courses.
Read More: IIT Roorkee to start School for Data Science and Artificial Intelligence
Instilling Right Mindset for Success
Sai Vignan advocates that data science for any product should not be a ‘wow’ factor but a need factor. Building anything attractive but not useful for the users will last only for a few days. Focus on building data science solutions that have long-term usability. Data science use-cases take a good amount of time to build. Companies do give the time needed but, if the data science leaders do not use it efficiently to build the right thing, time will be at stake for the product to continue in the market.
Therefore, he encourages building something that eases the user’s work and not that pleases the developer. He also suggests budding data scientists make decisions about their respective work/projects based on their statistical analysis instead of relying on a HIPPO (highest paid person’s opinion).