Amid the concerns that Moore’s law is grasping the last straws in the semiconductor industry, neuromorphic chips can be a revolutionary solution. Though silicon has blessed us in making computers take over the tech world, the limitations of miniaturization of silicon-Âbased transistors are finally catching up. Even artificial intelligence models need to go beyond the emulated classical logic and self-learning by perception for deriving reasoned conclusions. While neuromorphic computing is founded on the notion of replicating the function of the human brain, it has its own challenges too.
Researchers from Germany’s Max Planck Institute of Microstructure Physics and SEMRON GmbH have developed new energy-efficient memcapacitive devices (memory capacitors) that might be used to perform machine-learning algorithms. These devices, which were described in a study published in Nature Electronics, function by utilizing a charge shielding mechanism.
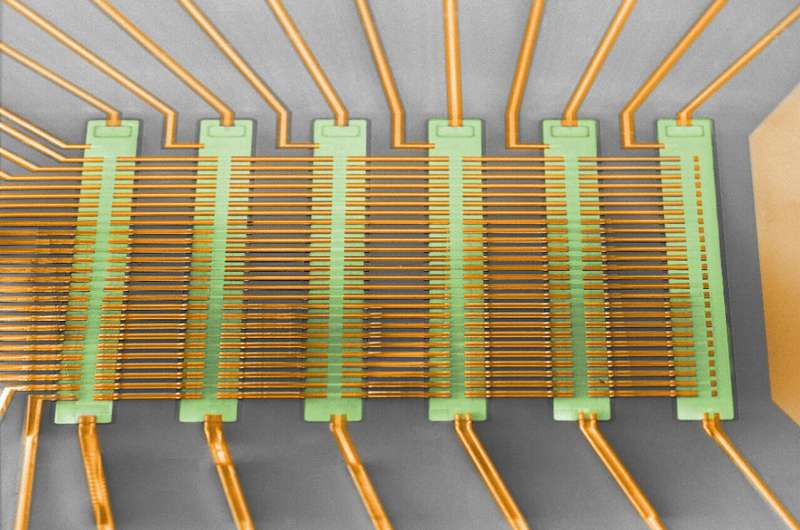
Memory circuit elements (memelements) are multidimensional electronic devices that have memory capability and whose resistance, capacitance, or inductance is determined by a previously applied voltage, charge, current, or flux. As a result, they mimic synapses, which are the connections between neurons in the brain, and whose electrical conductivity varies depending on how much electrical charge has traveled through them before.
Memristors, also known as memory resistors, are a type of electrical circuit building block that was hypothesized around 50 years ago but was developed for the first time a little more than a decade ago. Memcapacitive devices are related to memristive devices, however they work on a capacitive principle and may have lower static power consumption. In comparison, much research was not carried out using memcapacitive devices due to a lack of functional materials or systems whose capacitance can be controlled by external variables.
Neuromorphic computing aims to solve a wide range of computationally challenging jobs. Neuromorphic computers can do complicated calculations quicker, more effectively, and with a smaller footprint than classic von Neumann designs. These attributes provide strong motivations for building hardware that leverages neuromorphic architectures. The interest in designing such chips and devices is also backed by the possibility of augmenting the overall training and efficiency of artificial intelligence systems. Further, neural networks are getting more data-hungry than ever which directly influences the energy demands of training those models.
As per Kai-Uwe Demasius, one of the study’s researchers, the team discovered that, in addition to traditional digital ways for operating neural networks, there were largely memristive approaches and just a few memcapacitive suggestions. Not only that, the team also observed that all commercially available AI processors are digital/mixed-signal based, with only a few chips using resistive memory devices. This motivated them to look at a different technique based on a capacitive memory device.
Read More: Inside Intel’s Loihi 2 Neuromorphic Chip: The Upgrades and Promises
While examining previous research, Demasius and his colleagues have found that all existing memcapacitive devices were difficult to scale up and had a low dynamic range. As a result, they set out to create more efficient and scalable technologies. They drew inspiration for their innovative memcapacitive technology from synapses and neurotransmitters in the brain.
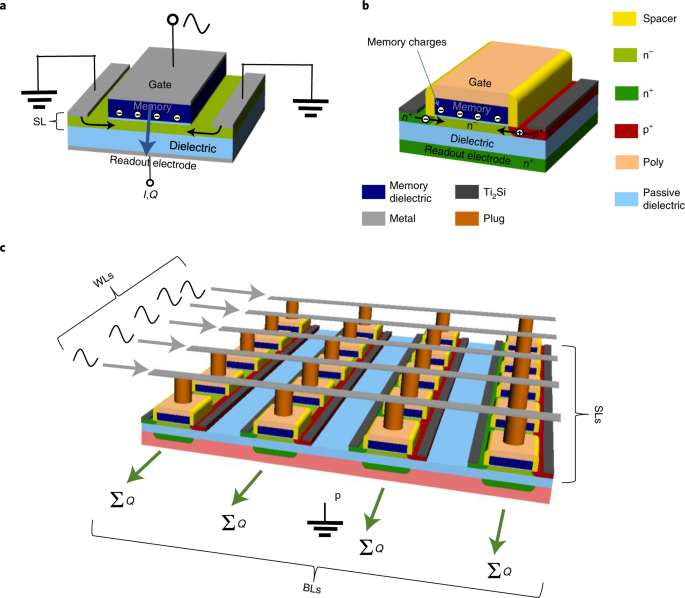
The researchers’ memcapacitive device has a top gate electrode, a shielding layer containing contacts, and a back-side readout electrode; these layers are segmented using dielectric layers. Here, the shielding layer can either transmit or strongly shield an electric field. An analog memory is used to alter this shielding layer by storing the varying weight values of artificial neural networks which is akin to how neurotransmitters in the brain store and transfer information. The top dielectric layer may have a memory effect, such as charge trapping or ferroelectricity, which may impact the shielding layer, or the shielding layer may have a memory effect.
Because memcapacitor devices are based on electric fields rather than currents, and have a greater signal-to-noise ratio, they are intrinsically several times more energy-efficient than memristive devices. Also, being based on charge screening, it allows for far greater scalability and a larger dynamic range than previous experiments on memcapacitive devices. Simply applying an external voltage to a doped junction or the influence of non-volatile memory in an upper dielectric layer can change the shielding layer’s properties.
To test the effectiveness of their memcapacitor devices, the researchers grouped 156 of them in a crossbar pattern, then used them to train a neural network to distinguish three different roman alphabet characters (“M,” “P,” and “I”). Their devices achieved astonishing energy efficiency of over 3,500 TOPS/W at 8 Bit precision, which is 35 to 300 times higher than other known memresistive techniques. These results show that the team’s novel memcapacitors have the ability to operate huge and complicated deep learning models with very low power consumption (in the μW regime).
As speech recognition software will go mainstream in the future, they will rely on the computing prowess of large neural network-based models with billions of parameters. Demasius and his colleagues are positive that their groundbreaking work in memcapacitor devices can usher a momentous impact in the future of artificial intelligence and neuromorphic applications. The team hopes to develop additional neural network-based models in the future, as well as scale up the memcapacitor-based system they created by boosting its efficiency and device density.